Pseudo RGB-D for Self-Improving Monocular SLAM and Depth Prediction
In European Conference on Computer Vision (ECCV), 2020
Authors: Lokender Tiwari1, Pan Ji2, Quoc-Huy Tran2, Bingbing Zhuang2, Saket Anand1, Manmohan Chandraker2,3
Affiliations: 1IIIT-Delhi, 2NEC Labs America, Inc., 3UCSD
Links: PDF, arXiv, Conference Talk and Demos, Conference Talk Slides, Demo Talk Slides,
Abstract
Classical monocular Simultaneous Localization And Mapping (SLAM) and the recently emerging convolutional neural networks (CNNs) for monocular depth prediction represent two largely disjoint approaches towards building a 3D map of the surrounding environment. In this paper, we demonstrate that the coupling of these two by leveraging the strengths of each mitigates the others shortcomings. Specifically, we propose a joint narrow and wide baseline based self-improving framework, where on the one hand the CNN-predicted depth is leveraged to perform pseudo RGB-D feature-based SLAM, leading to better accuracy and robustness than the monocular RGB SLAM baseline. On the other hand, the bundleadjusted 3D scene structures and camera poses from the more principled geometric SLAM are injected back into the depth network through novel wide baseline losses proposed for improving the depth prediction network, which then continues to contribute towards better pose and 3D structure estimation in the next iteration. We emphasize that our framework only requires unlabeled monocular videos in both training and inference stages, and yet is able to outperform state-of-the-art self-supervised monocular and stereo depth prediction networks (e.g., Monodepth2) and feature-based monocular SLAM system (i.e., ORB-SLAM). Extensive experiments on KITTI and TUM RGB-D datasets verify the superiority of our self-improving geometry-CNN framework.
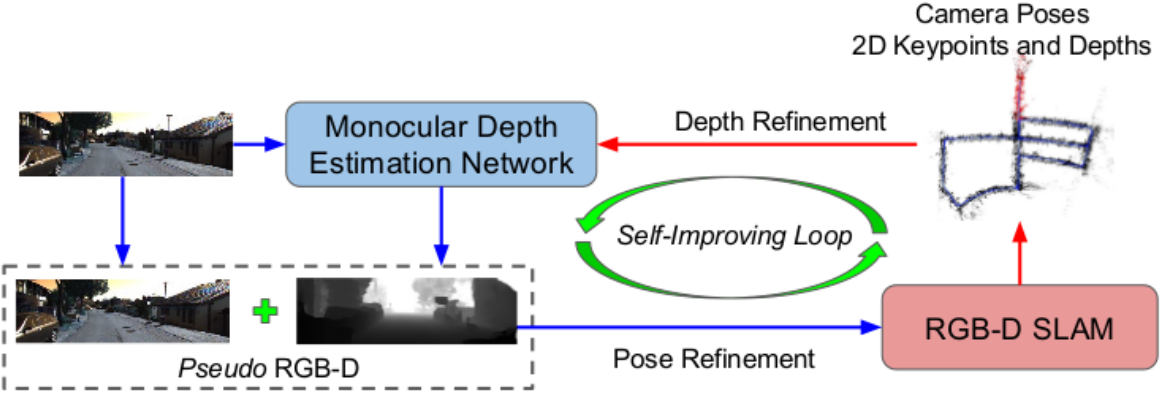
Figure 1: A SELF-SUPERVISED, SELF-IMPROVING FRAMEWORK -- It alternates between pose refinement (blue arrows) and depth refinement (red arrows).
ECCV 2020 Conference Talk
ECCV 2020 Demo Track : RGB vs Pseudo RGB-D SLAM and Monocular Depth Prediction Demos
Video 1: KITTI Odometry Sequence 19
Video 2: KITTI Odometry Sequence 11
Video 3: TUM RGB-D Sequence freiburg3 Large Cabinet Validation.
Results: Qualitative Depth Estimation
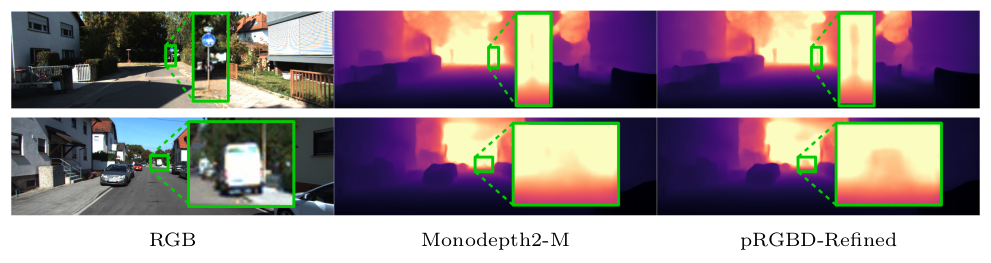
Figure 2: Qualitative depth evaluation results on KITTI Odometry test set. Improvement in depth prediction of farther scene points.
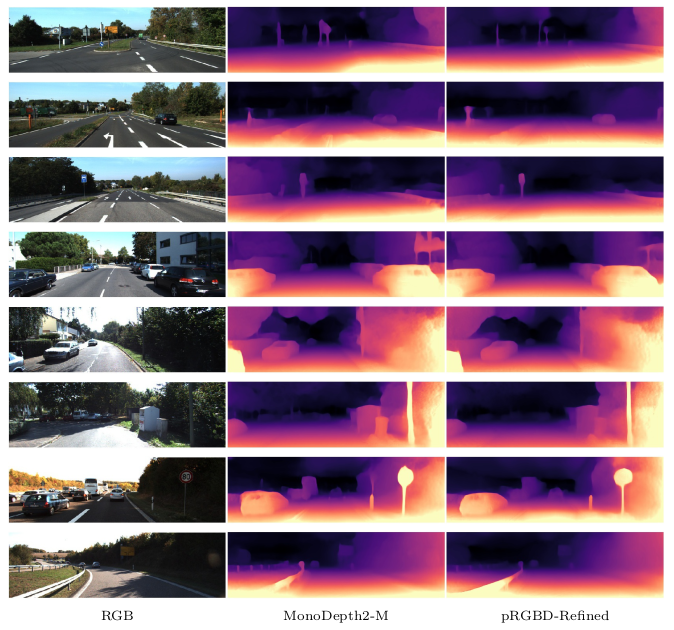
Figure 3: Qualitative depth evaluation results on KITTI Raw Eigen split test set. MonoDepth2-M is the MonoDepth2 model trained using monocular images.
Results: Qualitative Pose Estimation Results
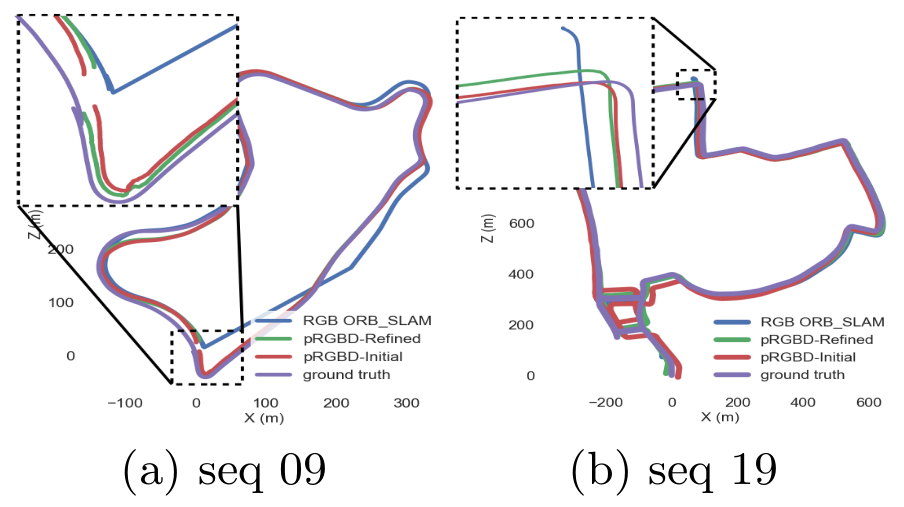
Figure 4: Qualitative pose evaluation results on KITTI Odometry sequences.
Bibtex
@inproceedings{tiwari2020pseudo, author={Tiwari, Lokender and Ji, Pan and Tran, Quoc-Huy and Zhuang, Bingbing and Anand, Saket and Chandraker, Manmohan}, title = {Pseudo RGB-D for Self-Improving Monocular SLAM and Depth Prediction}, booktitle = {European Conference on Computer Vision}, year = {2020} }